As usual, we will have a BoF (Birds of a Feather) session on Tuesday, Nov 16th, from 12:15pm to 1:15pm in Room 227-228. It is accessible for virtual attendees as well as exhibit attendees. NAMD, one of the earliest Charm++ applications, is featured in two of the six Gordon Bell award finalists in the special category aimed at COVID-19 research. Both papers will be presented in a session on Wednesday afternoon 1:30-3:00 pm in Room 240-242: Charmworks Inc. has a booth, #2030, where you can meet staff and researchers and see the fault tolerance and load balancing demo on our mini-cluster on site.
There are two papers at the ESPM2 workshop on Monday in Room 242 by researchers from UIUC and Charmworks with titles:
Accelerating Messages by Avoiding Copies in an Asynchronous Task-based Programming Model
Performance Evaluation of Python Parallel Programming Models: Charm4Py and mpi4py
Click to view our workshop website
The 19th Annual Workshop on Charm++ and Its Applications was held virtually on Oct 18-19, 2021. Please view the workshop website to see recordings of the talks and slides. The workshop is broadly focused on adaptivity in highly scalable parallel computing. It also takes stock of recent results in adaptive runtime techniques in Charm++ and the collaborative interdisciplinary research projects developed using it.
Click to view our workshop website
The 18th Annual Workshop on Charm++ and Its Applications will be held virtually on Oct 20-21, 2020. The workshop is broadly focused on adaptivity in highly scalable parallel computing. It also takes stock of recent results in adaptive runtime techniques in Charm++ and the collaborative interdisciplinary research projects developed using it. There will be keynotes as well as talks from external speakers, collaborators, and members of PPL.
Click to view our workshop website
The 17th Annual Workshop on Charm++ and Its Applications will be held on May 1-2, 2019, at the NCSA Auditorium. The workshop is broadly focused on adaptivity in highly scalable parallel computing. It also takes stock of recent results in adaptive runtime techniques in Charm++ and the collaborative interdisciplinary research projects developed using it. There will be talks from external speakers, including keynotes from Fred Streitz and Steve Plimpton.
Click to see our webpage for details regarding Charm++/AMPI at SC18
For Charm++, there will be a Bird of a Feather (BoF) session on Nov. 14th (Wed) 12:15 - 13:15 in D220. Charmworks, a startup that commercially supports Charm++ will be exhibiting in booth 3058. In addition, there will be a panel and a workshop keynote that are relevant to the Charm++ community.Click to see our webpage for BoF session details.
CharmPy takes advantage of features of the Charm++ runtime, including an object-based system, asynchronous remote method execution, automatic overlap of communication and computation, and dynamic load balancing.
Among the notable features in the recently released 0.10 version are support for Futures, threaded entry methods that facilitate direct-style programming, easy install via pip on Windows, macOS and regular Linux systems, and a Cython-based C-extension module for increased performance.
For more information on CharmPy, click here.
Charm++ is a machine independent parallel programming system. Programs written using this system run unchanged on MIMD machines with or without shared memory. It provides high-level mechanisms and strategies to facilitate the task of developing even highly complex parallel applications. Adaptive MPI is an MPI library implemented on top of Charm++, providing the high-level features of Charm++, such as dynamic load balancing and fault tolerance, to MPI applications.
For more information on the webinar, click here.



Prof. Kale was selected, alongside 53 others this year, to join the elite group of computer scientists that represents less than 1% of ACM's overall membership. ACM will formally recognize its 2017 Fellows at the annual Awards Banquet, to be held in San Francisco on June 23, 2018.
An excerpt from the Department of Computer Science news article "Kale, Zhai Named ACM Fellows" by David Mercer follows:
Kalé, who is the Paul and Cynthia Saylor Professor of Computer Science, was honored “for development of new parallel programming techniques and their deployment in high performance computing applications.”
He led pioneering work to develop adaptive runtime systems in parallel computing and incorporated it in the Charm++ parallel programming framework. Kale and his group are known for collaborative development of several highly scalable computational science and engineering applications, including NAMD (biophysics), ChaNGa (Astronomy), and OpenAtom (Quantum Chemistry).
Kalé also is a Fellow of the Institute of Electrical and Electronics Engineers, a winner of the 2012 IEEE Computer Society Sidney Fernbach Award, and a co-winner of the 2002 Gordon Bell Prize.
Q. What is the new parallel programming technique for HPC that you created?
A. I and my group have pioneered the idea of adaptive runtime systems in parallel computing, or HPC. We not only developed the ideas, but always incorporated them in a practical parallel programming system called Charm++ that we distribute and maintain from Illinois. We co-developed several science and engineering applications using Charm++, which allowed us to validate and improve the adaptive runtime techniques we were developing in our research in the context of full applications. The application codes developed include NAMD (biophysics), OpenAtom (quantum chemistry and materials modeling), ChaNGa (astronomy), Episimdemics (simulation of epidemics), and others.
Q. What HPC systems has it been deployed on, and where do you see it being deployed in the near future?
A. These are highly scalable codes that run from small clusters to supercomputers, including Blue, Waters, on hundreds of thousands of processor cores.
Q. What benefit does this bring to the larger HPC community?
A. Our approach allows parallel programmers to write code without worrying about where (on which processor) the code will execute, or which data will be on what processor.
The runtime system continuously watches the program behavior and moves data and code-execution among processors so as to automatically improve performance, via dynamic load balancing. This approach especially helps development of complex or sophisticated parallel algorithms.
Q. Becoming an ACM Fellow is obviously quite an honor. Tell me a bit about your career path and how you believe it led you to this point.
A. Apart from the novelty of automated runtime optimization, my research is characterized by continuous engagement with science applications. This is a costly path for a researcher, and I paid the price for it early in my career. But I believed such application-oriented yet computer science-centered research is the only way to try to make a lasting impact.
The credit for my success and for this award certainly goes to generations of my students who worked on various aspects of adaptive runtime systems.
ACM's announcement can be found here.
Congratulations Prof. Kale!

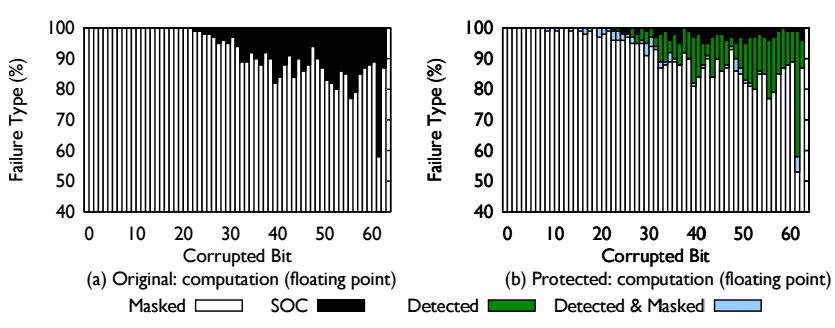
The decreasing size of transistors has been critical to the increase in capacity of supercomputers. The smaller the transistors are, less energy is required to flip a bit, and thus silent data corruptions (SDCs) become more common. In this paper, we present FlipBack, an automatic software-based approach that protects applications from SDCs. FlipBack provides targeted protection for different types of data and calculations based on their characteristics. It leverages compile-time analysis and program markup to identify data critical for control flow and enables selective replication of computation by the runtime system. We evaluate FlipBack with various HPC mini-applications that capture the behavior of real scientific applications and show that FlipBack is able to protect applications from most silent data corruptions with only 6 − 20% performance degradation.
We have extended OpenAtom to support several interesting ab-initio molecular dynamics simulation methods, in addition to the Car-Parrinello method and Born-Oppenheimer method, including k-points, parallel tempering, and path integrals. Recent technical advances in OpenAtom include the construction of a generic library for calculating FFT's in charm with minimal communication, Charm-FFT, the inclusion of generalized topology-aware mapping schemes, and the ability to run "Uber" or multi-instance methods in a single run.
The following graph highlights recent performance results we obtained on Blue Gene/Q and Cray XE6/XK7 systems using a variety of the methods newly integrated into OpenAtom. These include topology-aware mapping and showcase Charm++'s unqiue suitability to this problem.
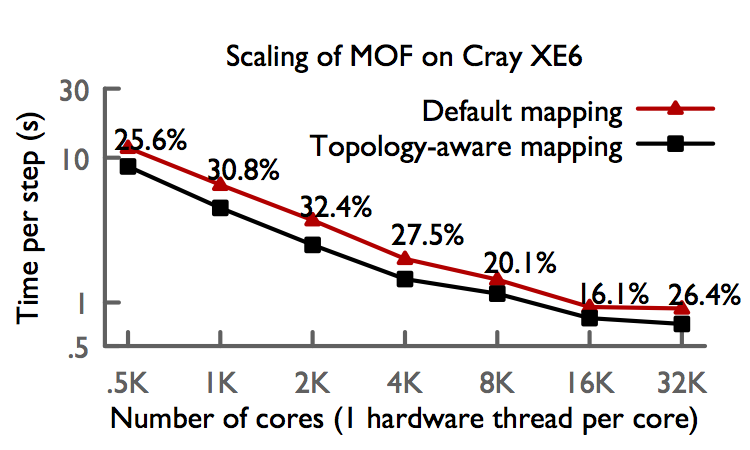

The design and manufacture of present-day CPUs causes inherent variation in supercomputer architectures such as variation in power and temperature of the chips. The variation also manifests itself as frequency differences among processors under Turbo Boost dynamic overclocking. This variation can lead to unpredictable and suboptimal performance in tightly coupled HPC applications. In this study, we use compute-intensive kernels and applications to analyze the variation among processors in four top supercomputers: Edison, Cab, Stampede, and Blue Waters.
As shown in the below figures, we observe that there is an execution time difference of up to 16% among processors on the Turbo Boost-enabled supercomputers: Edison, Cab, Stampede. There is less than 1% variation on Blue Waters, which does not have a dynamic overclocking feature. We analyze measurements from temperature and power instrumentation and find that intrinsic differences in the chips’ power efficiency is the culprit behind the frequency variation.

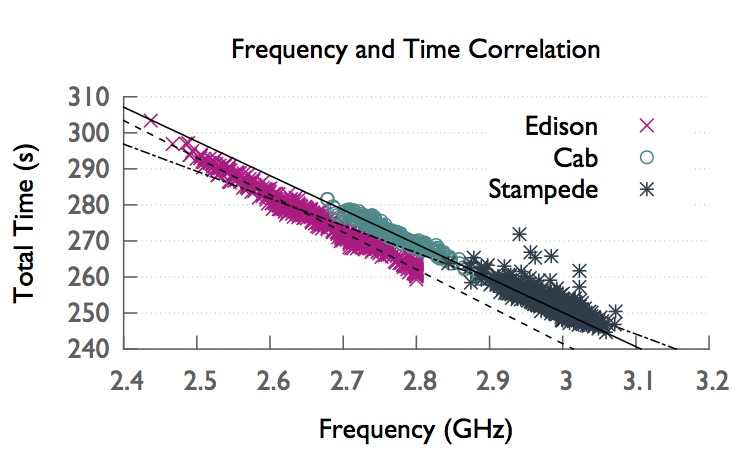
Moreover, we show that with a speed-aware dynamic load balancing algorithm we can reduce the negative effects of performance variation and improve the performance up to 18% compared to no load balancing performance and 6% better than the non-speed-aware counterpart.
For more details, please read ICS'16 paper: http://charm.cs.illinois.edu/newPapers/16-08/paper.pdf
and VarSys, IPDPS'16 paper: http://charm.cs.illinois.edu/newPapers/16-03/paper.pdf


Parallel Discrete Event Simulation (PDES) is a type of simulation that differs significantly from many common HPC applications. In PDES, the simulation progresses forward by processing events that happen at discrete points in virtual time. The distribution of these events in time, as well as how the events affect the state of the simulation, may result in a very irregular pattern of communication that is not known a priori. Due to the dynamic and irregular nature of PDES, we believe that Charm++ makes a good target for implementing a scalable PDES engine. Over the past two years we have collaborated with the ROSS (http://www.cs.rpi.edu/~chrisc/ross.html) team at RPI to reimplement ROSS on top of Charm++. ROSS is a PDES engine that has been developed and highly optimized on top of MPI. ROSS has shown great scalability when running models with a low amount of inter-process communication, however it has shown some limitations for more communication intensive models. Because the Charm++ runtime system is able to effectively handle asynchronous communication, overlapped automatically with computation, the Charm++ version of ROSS is able to overcome some of these limitations. As shown in the plot below, as we increase the amount of inter-process communication, the Charm++ version of ROSS achieves up to 40% higher event rates than the MPI version of ROSS on up to 2 million cores. More details are in the PADS’16 paper (http://charm.cs.illinois.edu/papers/16-01).
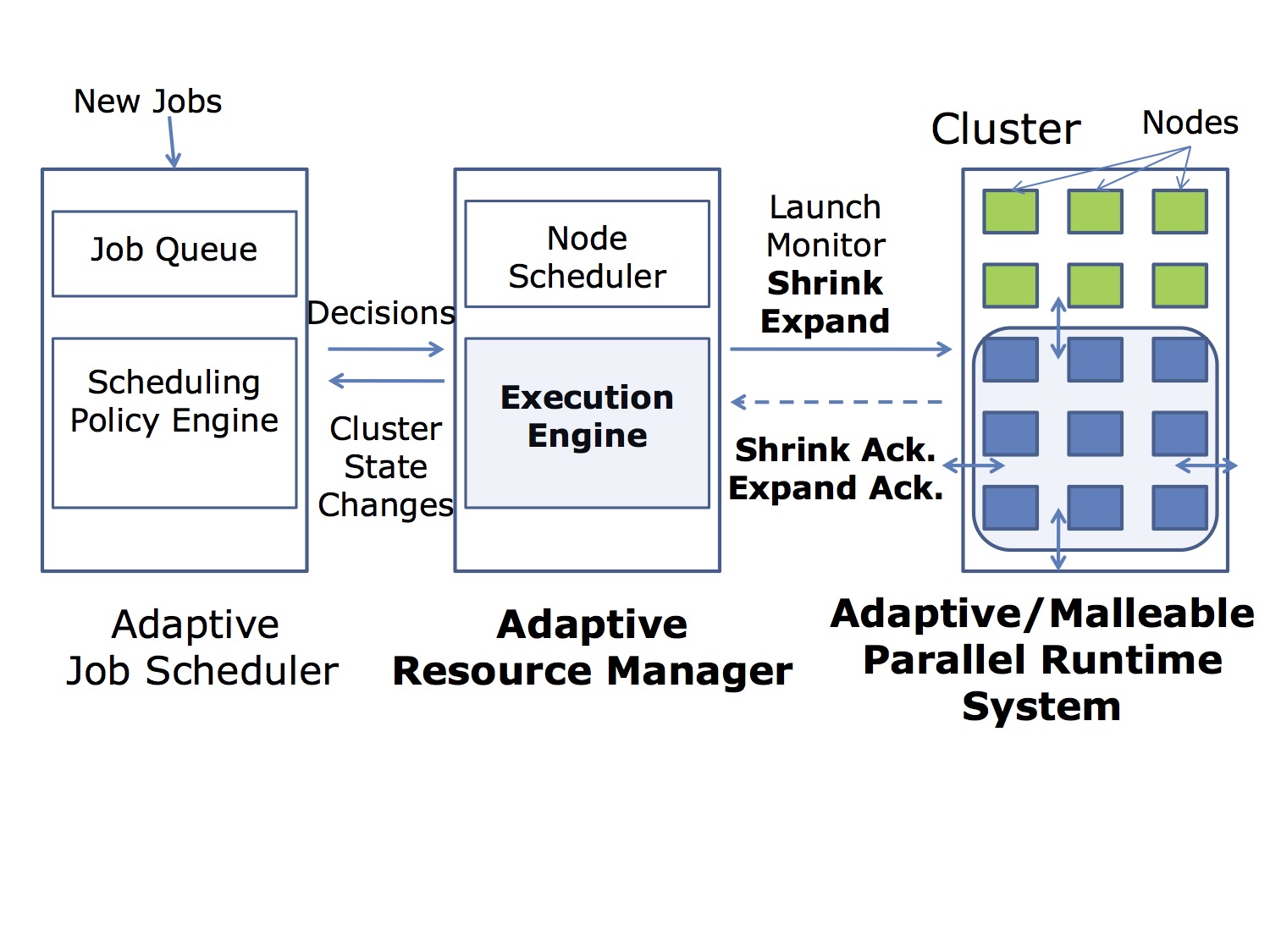
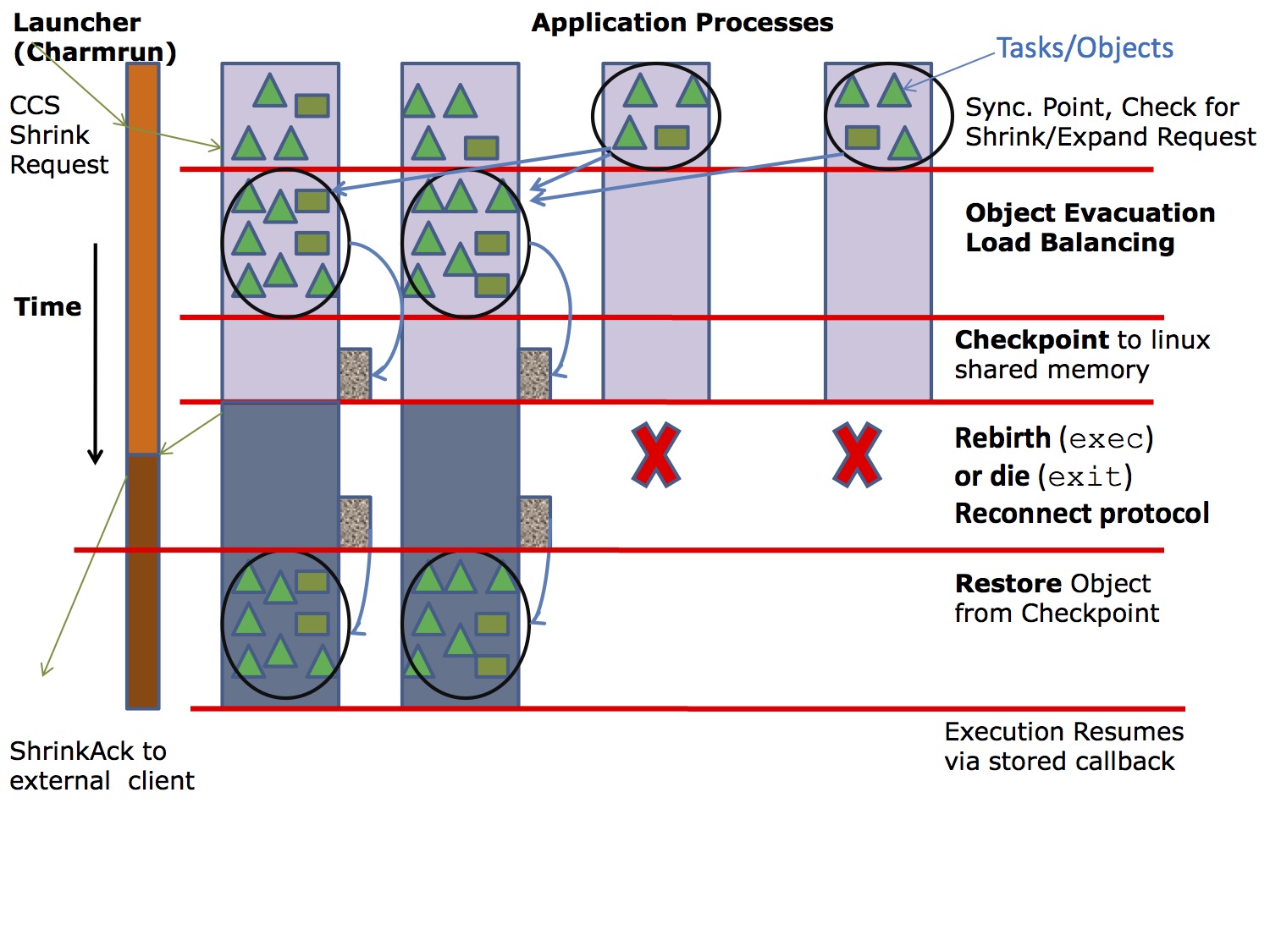
We add shrink and expand capability to Charm++ adaptive parallel runtime system and show the efficacy, scalability, and benefits of our approach, which is based on task migration and dynamic load balancing, checkpoint-restart, and Linux shared memory. Shrinking from 2k to 1k cores takes 16s while expand from 1k to 2k takes 40s. Figures below show the performance of rescaling with difference problem size and different number of processors.
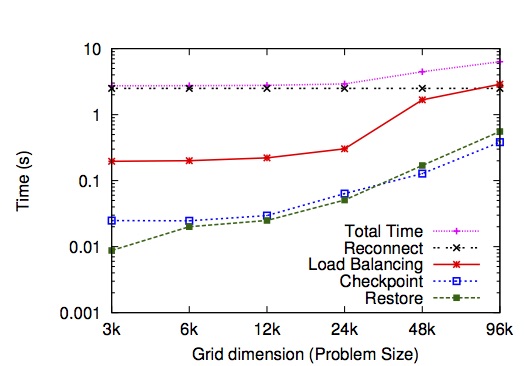

In our later collaboration, we show an implementation of an adaptive resource manager by making Torque/Maui malleable with a malleable job-scheduling algorithm and show the integration with Charm++. For more details on Charm++ implementation of malleability, see our HiPC’14 paper: http://charm.cs.uiuc.edu/papers/14-29 For more details on malleability with Torque/Maui see our IPDPS’15 paper: http://charm.cs.uiuc.edu/papers/15-12
We use the term logical structure to denote an exact ordering of events reflecting patterns of parallel dependency behavior as de- signed by the application’s developers. Generally, programming such applications requires breaking the problem into smaller pieces, or phases, some of which require parallel interaction. The logical structure separates these phases which may be interleaved in physical time. Similarly, within each phase, the events are ordered by their dependencies into discrete logical steps rather than their true physical time order and displacement. This matches how the events were programmed: with logical ordering rather than known timing information guiding the design.
In the above figure, we show the logical and physical timeline of the Jacobi2d application. In the logical view, the reasons for the idle time experienced in the application become more evident: namely a reduction that is mapped to processors directly.
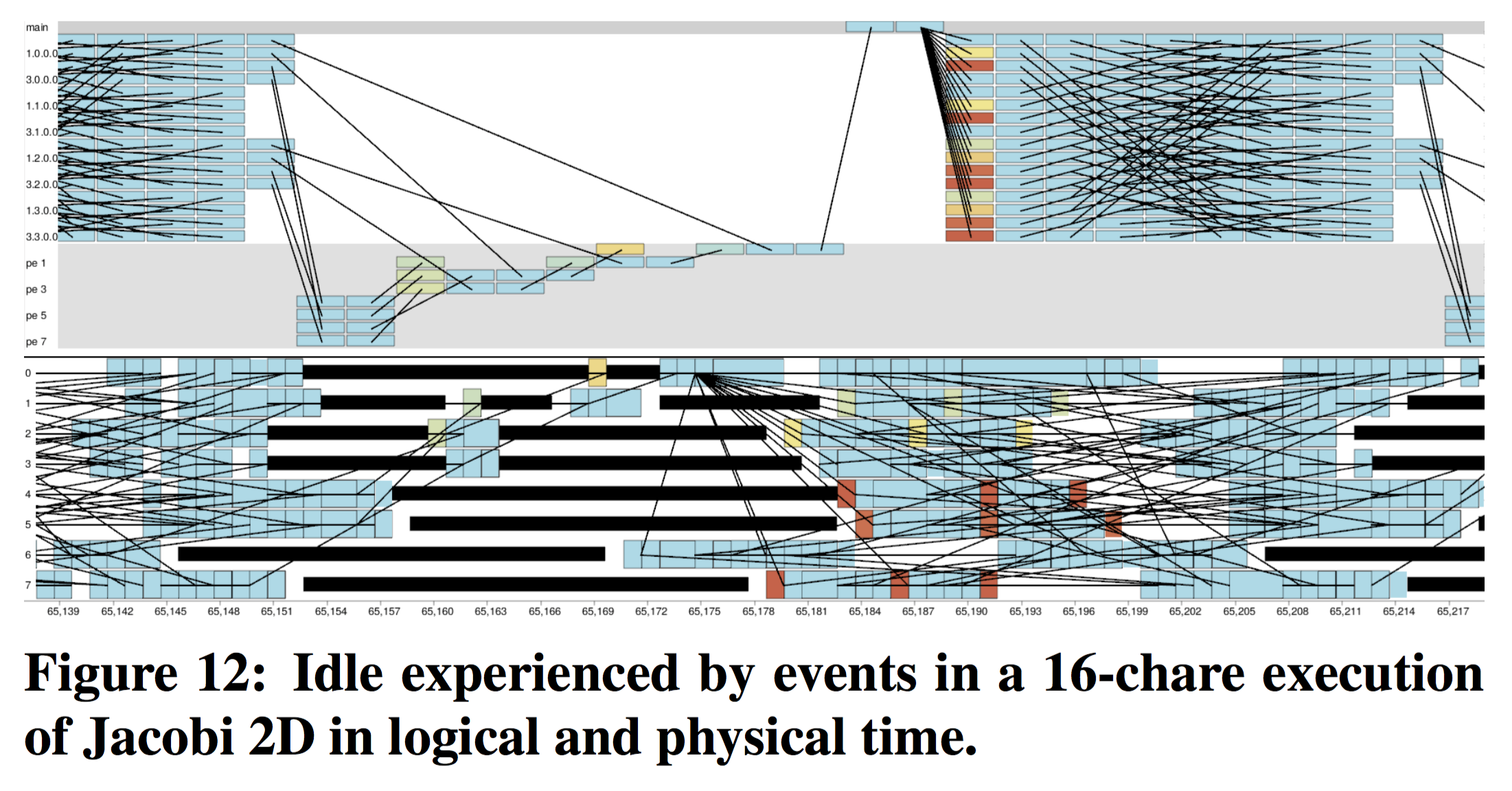
TraceR is a trace replay tool built upon the ROSS-based CODES simulation framework. It executes under an optimistic parallel discrete-event paradigm using reversible computing for production HPC applications. It can be used for predicting network performance and understanding network behavior by simulating messaging on interconnection networks. It addresses two major shortcomings in current network simulators. First, it enables fast and scalable simulations of large-scale supercomputer networks. Second, it can simulate production HPC applications using BigSim's emulation framework.
Figure below presents the execution time for simulating 3D Stencil on various node counts of 3D torus. TraceR scales well for all simulated system sizes. For the simulations with half a million (512K) nodes, the execution time is 542 seconds using 3D TorusNet model.

We also do a validation study with LeanMD application on 5D Torus. We compare real execution time with simulated execution time in Figure below. We observe that for all node counts (512 to 8192nodes) the error in the prediction is less than 9%. For most cases, the predicted time is within 2% of the observed execution time.

For more details: http://charm.cs.illinois.edu/newPapers/15-14/paper.pdf
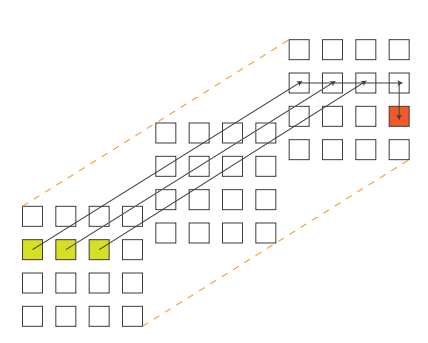
It is often convenient to use fine-grained messages to implement the interactions between objects in a Charm++ program, because the typically small work and data units that result from object-based decomposition tend to produce small message payloads. While the utility of fine-grained communication is clear, its performance characteristics are poor due to overhead. Much of the per message overhead at the application, RTS, and network level is independent of message size. In applications with large fine-grained message volume, this overhead can dominate run time. The Charm++ Topological Routing and Aggregation Module (TRAM) is a library that improves fine-grained communication performance by coalescing fine-grained communication units, or data items, into larger messages. TRAM constructs a virtual topology comprising the processes in the parallel run, and defines peers within this topology as any processes that can be reached by traveling an arbitrary number of hops along a single dimension. Separately aggregating data items for each destination requires substantial memory and limits the potential for aggregating concurrent data items with different destinations but common sub-paths. To address this issue, TRAM aggregates data items at the level of peers. Items whose destinations are not in the set of peers at the source process are routed through one or more intermediate destinations along a minimal route. As a result, the memory footprint of the buffer space normally fits in lower level cache.
TRAM can dramatically improve performance for applications dominated by fine-grained communication. An example is the simulator of contagion, EpiSimdemics, where TRAM provides up to a 4x speedup compared to direct use of fine-grained messages. In strong scaling scenarios for EpiSimdemics, TRAM also allows for better scaling than an application-specific point to point aggregation approach. As the number of nodes in a run is increased, the individual sends are distributed throughout the network, so that direct aggregation at the source for each destination fails to aggregate items into large enough buffers to sufficiently mitigate communication overhead. By comparison, a topological aggregation approach is able to maintain the advantage of aggregation to much higher node counts.
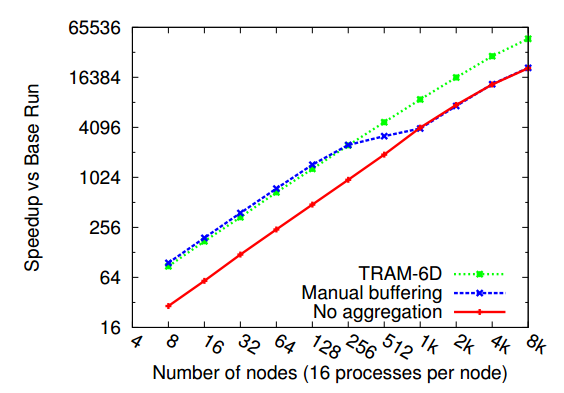
Link to thesis.
ICPP paper.
SC paper.
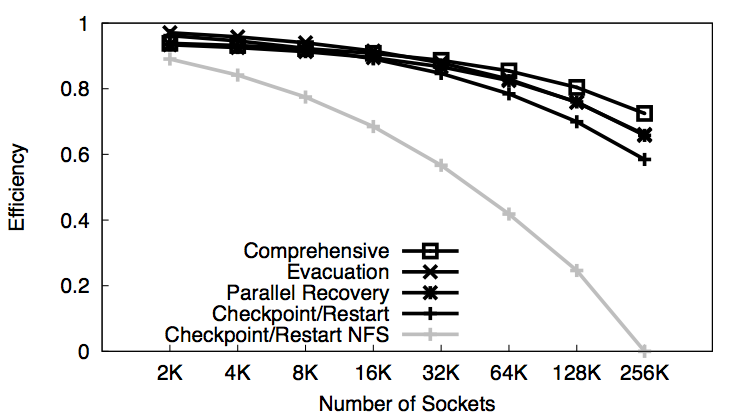
It has been long recognized by the HPC community that reliability is one of the major hurdles in reaching extreme scale. The same gigantic number of components that will make supercomputers powerful, will make them equally vulnerable to frequent failures. Estimates differ as to how often extreme scale machines will crash, but there is a consensus that applications will require some form of fault tolerance.
Migratable objects facilitate the task of developing new resilient algorithms, or refining existing ones. The very nature of the programming model, on which Charm++ is based, allows more flexibility in the execution of an application. That same flexibility is crucial in adapting to failures.
In a recent journal publication, we collected our work on fault tolerance strategies using migratable objects. The figure shows the efficiency of those strategies when projected at extreme scale. The traditional checkpoint/restart based on a network file system will not scale too far. However, schemes enriched with migratable objects may keep the efficiency of extreme-scale systems above 60%. Among those schemes we find checkpoint/restart based on local storage, proactive evacuation, and parallel recovery with message logging. A comprehensive approach, combining all the schemes, may increase the efficiency of a system to almost 80% at extreme scale.
Link to IEEE Transactions on Parallel and Distributed Systems publication
Link to research area page on Fault Tolerance
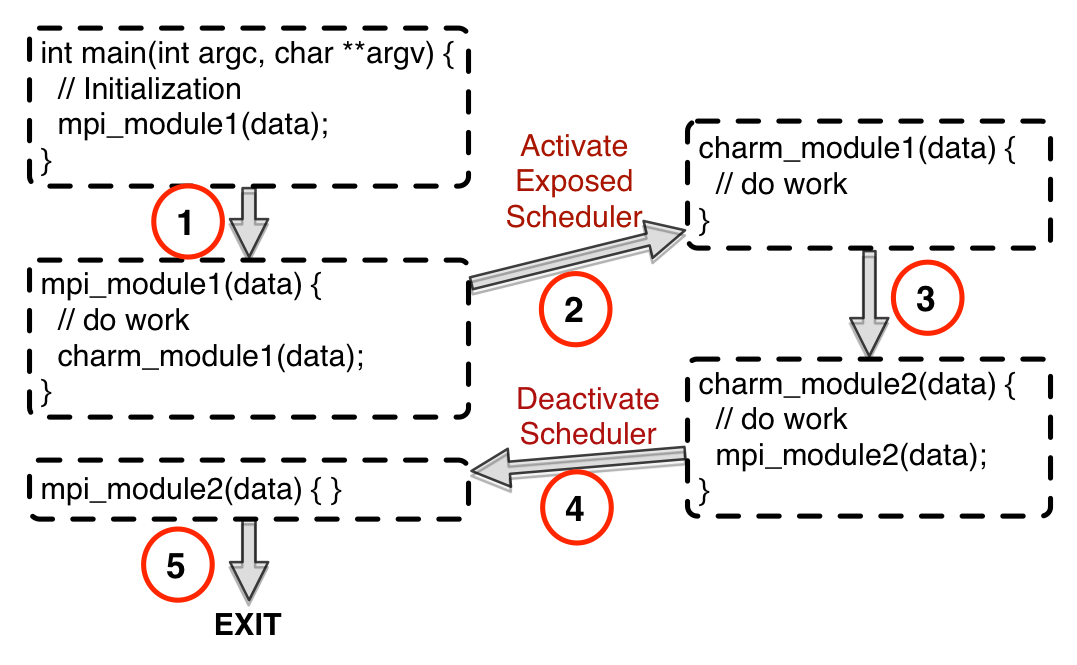
This work explores a framework that enables hybrid parallel programming with MPI and Charm++, and allows programmers to develop different modules of a parallel application in these two languages while facilitating smooth interoperation. MPI and Charm++ embody two distinct perspectives for writing parallel programs. While MPI provides a processor-centric, user-driven model for developing parallel codes, Charm++ supports work-centric, overdecomposition-based, system-driven parallel programming. One or the other can be the best or most natural fit for distinct modules that constitute a parallel application. In the published work linked below, we describe the challenges in enabling interoperation between MPI and Charm++, and present techniques for managing the control flow and resource sharing in such scenarios. As the attached figure indicates, interoperation between MPI and Charm++ has been enabled by exposing the Charm++ scheduler to the programmer, and enabling the reuse of Charm++ and MPI applications as external libraries. We also demonstrate the benefits of interoperation between MPI and Charm++ through several case studies that use production applications and libraries, including CHARM/Chombo, EpiSimdemics, NAMD, FFTW, MPI-IO and ParMETIS.
Link to the paper published in IPDPS, 2015.
Link to the presentation at IPDPS, 2015.
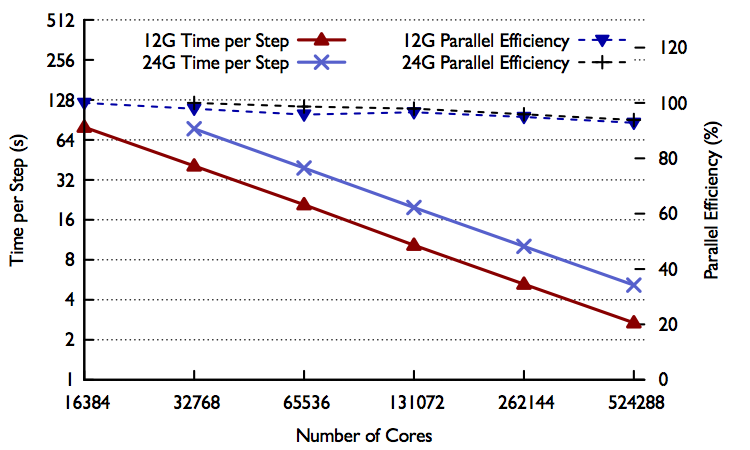
Simulating the process of cosmological structure formation with enough resolution to determine galaxy morphologies requires an enormous dynamic range in space and time. ChaNGa is a parallel n-body+SPH cosmology code implemented using Charm++ for the simulation of astrophysical systems on a wide range of spatial and time scales. Scaling such codes to large processor count requires overcoming not only spatial resolution challenges, but also large ranges in timescales. In this work, we use an approach called multi-stepping which involves using different time steps for different particles in relation to their dynamical time scales, leading to an algorithm that is challenging to parallelize effectively. We present the optimizations implemented in ChaNGa that allow it to scale to large numbers of processors, and address the challenges brought on by the high dynamic ranges of clustered datasets. We demonstrated strong scaling results for uniform datasets on up to 512K cores on Blue Waters evolving 12 and 24 billion particles (Fig 1). We also have shown strong scaling results for cosmo25 and dwarf datasets, which are more challenging due to their highly clustered nature. In Fig 2, we can see that we obtain good performance on up to 128K cores of Blue Waters and also show up to a 3 fold improvement in time with multi-stepping over single-stepping.
Link to the paper: PDF
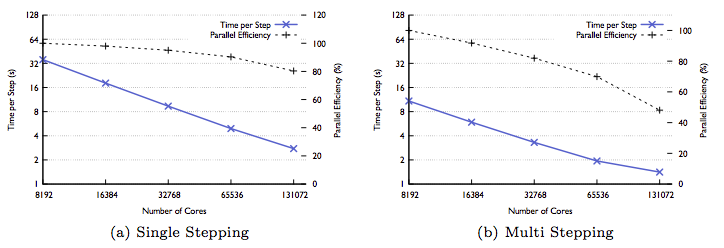
Asynchronous Contact Mechanics (ACM) algorithm is a reliable method to simulate flexible material subject to complex collisions and contact geometries. For example, we can apply ACM to perform cloth simulation for animation. The parallelization of ACM is challenging due to its highly irregular communication pattern, its need for dynamic load balancing, and its extremely fine-grained computations. In our recent work, we utilize CHARM++ to address these challenges and show good strong scaling of ACM to 384 cores for problems with fewer than 100k vertices. By comparison, the previously published shared memory implementation only scales well to about 30 cores for the same examples. We demonstrate the scalability of our implementation through a number of examples which, to the best of our knowledge, are only feasible with the ACM algorithm. In particular, for a simulation of 3 seconds of a cylindrical rod twisting within a cloth sheet, the simulation time is reduced by 12× from 9 hours on 30 cores to 46 minutes using our implementation on 384 cores of a Cray XC30.
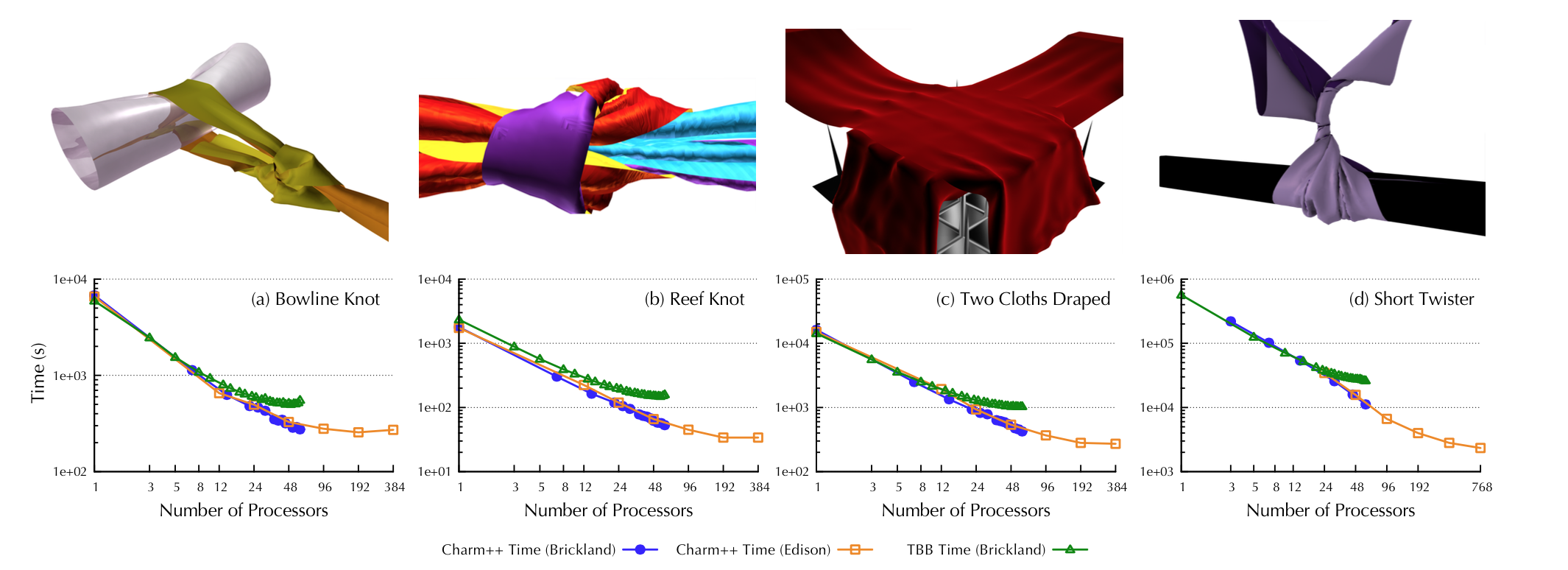
For more details, please refer to the IPDPS'2015 publication here.
Recent advances in processor and memory hardware designs have made it possible for the user to control the power consumption of the CPU and memory through software, e.g., the power consumption of Intel’s Sandy Bridge family of processors can be user-controlled through the Running Average Power Limit (RAPL) library. It has been shown that increase in the power allowed to the processor (and/or memory) does not yield a proportional increase in the application’s performance. As a result, for a given power budget, it can be better to run an application on larger number of nodes with each node capped at lower power than fewer nodes each running at its TDP. This is also called overprovisioning. The optimal resource configuration for an application can be determined by profiling an application’s performance for varying number of nodes, CPU power and memory power and then selecting the best performing configuration for the given power budget. In this paper, we propose a performance modeling scheme that estimates the essential power characteristics of a job at any scale. Our online power aware resource manager (PARM) uses these performance characteristics for making scheduling and resource allocation decisions that maximize the job throughput of the supercomputer under a given power budget. With a power budget of 4.75 MW, PARM can obtain up to 5.2X improvement in job throughput when compared with the SLURM scheduling policy that is power-unaware. With real experiments on a relatively small scale cluster, PARM obtained 1.7X improvement. An adaptive runtime system allows further improvement by allowing already running jobs to shrink and expand for optimal resource allocation. Link to the paper: PDF



Figure 1(a,b) show the best cache configurations and the energy savings using our method for different problem sizes of HPCCG application. As illustrated, our approach can save significant energy when the problem size is either smaller or larger than the cache sizes.

This work, accepted to SC'14, analyzes the behavior of the dragonfly network for various routing strategies, job placement policies, and application communication patterns. The study is based on a novel model that predicts traffic on individual links for direct, indirect, and adaptive routing strategies. In the paper, we analyze results for single jobs and some common parallel job workloads. The predictions presented in this paper are for a 100+ Petaflop/s prototype machine with 92,160 high-radix routers and 8.8 million cores.
The general observations are that a randomized placement at the granularity of nodes and routers and/or indirect routing can help spread the messaging traffic over the network and reduce hot-spots. If the communication pattern results in non-uniform distribution of traffic, adaptive routing may provide significantly better traffic distributions by reducing hot-spots. For parallel job workloads, adaptive hybrid routing is useful for combining good features of adaptive direct and adaptive indirect routings and may provide a good traffic distribution with lower maximum traffic. Adaptive routings also improve the traffic distribution significantly in comparison to static routings. We also observed that allowing the users to choose a routing for their application can be beneficial in most cases on dragonfly networks. Use of randomized placement at the granularity of nodes and routers is the suggested choice for such scenarios also.
Paper link on PPL's website
Paper schedule at SC'14
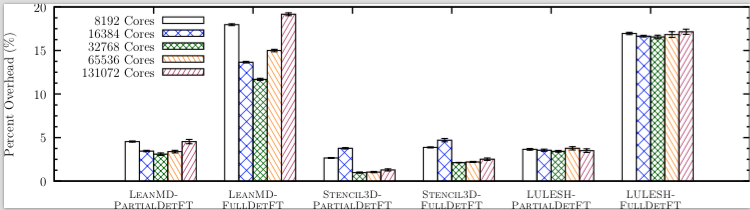
Fault tolerance has become increasingly important as system sizes become larger and the probability of hard or soft errors increases. In this work, we focus on methodologies for tolerating hard errors: those that stem from hardware failure and cause a detectable fault in the system. Many approaches have been applied in this area, ranging from checkpoint/restart, where the application's state is saved to disk, to in-memory checkpoint, where the application incrementally saves its state to partner nodes. Both of the approaches, while effective, require the entire system to synchronize and rollback to the checkpoint to ensure consistent recovery. Message logging is an effective methodology for saving the messages that were sent in a localized manner, along with there order, which is encoded in a determinant. Message logging is also effective for deterministic replay, which is commonly used for discovering bugs.
For message passing programs, a major source of overhead during forward execution is recording the order in which messages are sent and received. During replay, this ordering must be used to deterministically reproduce the execution. Previous work in replay algorithms often makes minimal assumptions about the programming model and application to maintain generality. However, in many applications, only a partial order must be recorded due to determinism intrinsic in the program, ordering constraints imposed by the execution model, and events that are commutative (their relative execution order during replay does not need to be reproduced exactly). In a paper published at CLUSTER'14 (to be presented in Madrid, Spain in September), we present a novel algebraic framework for reasoning about the minimum dependencies required to represent the partial order for different orderings and interleavings. By exploiting this framework, we improve on an existing scalable message-logging fault tolerance scheme that uses a total order. The improved scheme scales to 131,072 cores on an IBM BlueGene/P with up to 2x lower overhead.
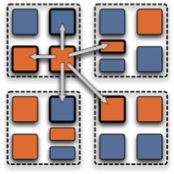
Science and engineering applications are increasingly becoming complex. Further, we are faced with new challenges due to dynamic variations in the machine itself. In the face of these challenges, what are the key concepts that are essential for parallel applications to take advantage of today’s modern supercomputers? In the paper accepted at SC14, we describe key designs and attributes that have been instrumental in enabling efficient parallelization. The paper describes the state of practice of CHARM++, using multiple mini-applications as well as some full-fledged applications and showcase various features enabled by the execution model.
It’s becoming more and more apparent that adaptive run time systems (RTS) will play an essential role in addressing various challenges in scaling parallel applications. However, to support the RTS, the programming model must possess certain key attributes including over-decomposition, asynchronous message driven and migratability. Over decomposition along with support for migratability can be exploited by the run time system to enable critical features and perform adaptive intelligent strategies. Over decomposition allows the run time system to map and remap objects to processors at run time which can be exploited to enable features such as adaptive load balancing, automatic checkpoint and restart, thermal and power management, adaptive job scheduling and communication optimizations. With migratability, the run time system can perform dynamic load balancing by migrating objects from overloaded processors to underloaded ones and adapt based on the application characteristics. It can also be used to automatically checkpoint the objects and restart them on a different number of processors. The shrink and expand feature also uses this capability to improve the cluster utilization by adaptively changing the number of processors assigned to a job and migrating the corresponding objects. We can use DVFS for temperature control and dynamic load balancing to decrease the cooling energy. The run time system can also monitor the communication pattern and with the knowledge of the underlying topology performs various communication optimizations. We show the benefits of these attributes and features on various mini-apps and full-scale applications run on modern day supercomputers.